Thoughts on the Inauguration of the Trustworthy and Responsible AI Lab by Axa and Sorbonne University
03 May 2022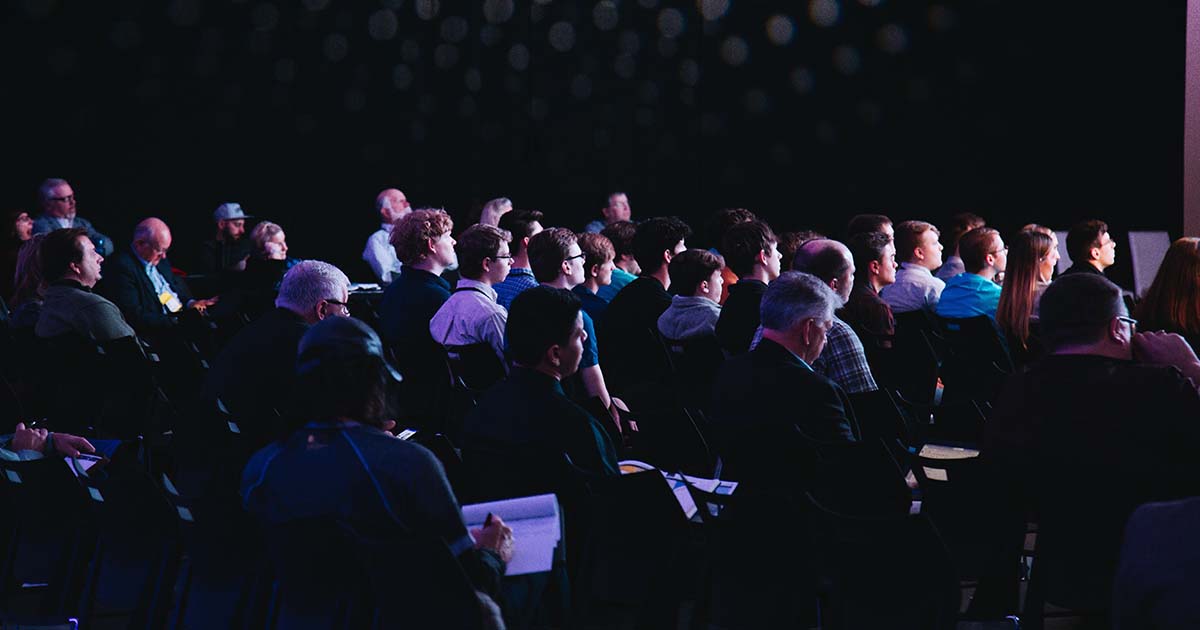
by Joshua Brand and Dilia Carolina Olivo from Operational AI Ethics (OpAIE), Télécom Paris
The event was headlined by two keynote presentations by Joanna Bryson and David Martens.
Talk from Prof. Joanna Bryson: AI is Necessarily Irresponsible
Impressions by Joshua Brand
Dr. Bryson’s presentation, provocatively entitled AI is Necessarily Irresponsible, introduced a foundational problem to the implementation of AI. At its current stage, AI is not itself capable of explaining or justifying its own actions. Given this lack of self-explainability, AI cannot be attributed with moral agency — this form of agency refers to those who have the power to decide on and pursue a course of action, and can be held accountable for such actions. Consequently, AI ought to be considered, not as irresponsible as the title suggests, but as aresponsible, or the inability to ever be responsible. Dr. Bryson subsequently tasked us with thinking about how we ought to move forward in light of this aresponsibility. She reminds us that any society, organized and held together by a set of values, needs to maintain responsibility for actions and subsequently, hold people accountable should we deem their actions wrong. We should thus pressure the companies who develop and implement AI to make it transparent and explainable to help promote accountability. This openness will then help to influence the developers, owners, and users of AI to ensure that its algorithmic outputs better align with the values of our societies. Dr. Bryson then completed her presentation with practical suggestions, such as ensuring that detailed logs are kept on changes to code and for each test performed before and during its release.
The enduring question I have after listening to Dr. Bryson’s presentation surrounds the definition of moral agency — an essential concept when attributing responsibility and accountability. As any philosopher knows, defining moral agency is not an easy endeavour particularly when we consider the free will-determinism debate on what causes us to act [1]. A common theory known as compatibilism accepts that people have autonomy and the ability to choose and cause their own actions to a certain degree, but nevertheless genetic and societal factors can evade our autonomy and influence our actions [2]. In this case, attributing responsibility is still possible, but can be complex. For example, should we hold a developer fully accountable for an algorithm if we cannot determine whether the developer was compelled to act by external forces? This problem is further exacerbated when we consider how many actors are involved with the development, deployment, and use of AI. It gets difficult in deciding who exactly is to blame for ethically faulty AI. While I do not consider this to be detrimental to Dr. Bryson’s presentation, it shows the difficult, but necessary, road ahead if we continue to develop and expand our use of AI.
1. This is a widely discussed and nuanced debate in philosophy, for an overview see Section 1 in Matthew Talbert, “Moral Responsibility”, The Stanford Encyclopedia of Philosophy, Winter 2019, accessed April 9, 2022
2. For a further look into compatibilism, see Michael McKenna and D. Justin Coates, “Compatibilism”, The Stanford Encyclopedia of Philosophy, Fall 2021, accessed April 9, 2022
Talk from Prof. David Martens: The counterfactual explanation: yet more algorithms as a solution to explain complex models?
Impressions by Dilia Carolina Olivo
Martens’ talk, titled « The counterfactual explanation: yet more algorithms as a solution to explain complex models? » invites us to think beyond feature importance for algorithmic explainability. He proposes a counterfactual method to explain algorithmic decisions by analyzing the changes required to affect an individual prediction.
For example, when an individual client wants to understand the decision made by the company, their bank teller could use counterfactual explanations to show that the model *would have* made a different prediction *if* certain input characteristics were different. Martens makes the observation that these kinds of explanations are useful for « unfair decisions » – when a client is unhappy with a decision, they want to know why *their* outcome was unfavorable compared to others (if the algorithm’s decision is favorable for the client, they likely will not be questioning it), hence the utility of using counterfactuals to compare the clients profile to hypothetical profiles with favorable outcomes.
Of course, the drawback of counterfactual methods is that the explanations given are only local, and cannot be extrapolated to the whole model.
Depending on the context for which these explanations are needed, the local nature of counterfactual explanations might not be an issue – on the contrary, for a hypothetical client looking to understand one decision, it is more appropriate to be direct than to overload the client with information. However for a regulatory body that wants to make sure that the algorithm respects regulatory standards, local explanations are not enough.
In reality, a mix of existing global and local methods are necessary in order to more closely respect explainability requirements in industry.