More AI, and less box-ticking, says FATF in AML/CFT report
13 July 2021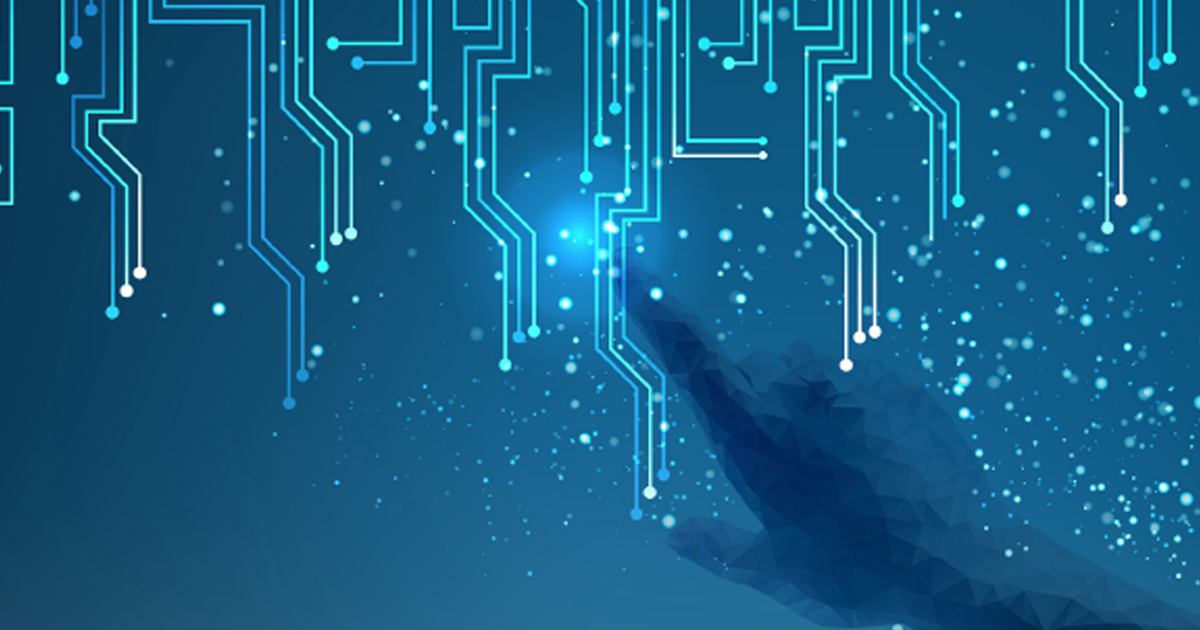
AI helps financial inclusion
AI can help improve financial inclusion by allowing financial institutions to be more precise in their onboarding risk analysis. Current methods of customer risk analysis are too rule-based, according to the FATF, leading to exclusion of broad groups of customers considered risky. Some of these excluded groups also are the most vulnerable, and have the most need to access financial services. According to the FATF, better access to financial services for individuals from risky groups has been held back both by unwillingness to make full use of the flexibility offered under the risk-based approach, and also by profit-based business decisions of financial institutions. By combining digital ID solutions and more precise AI-based transaction monitoring, financial institutions can accept customers that might not otherwise have been able to clear the initial customer due diligence (CDD) risk assessment. AI can facilitate behavioral and contextual risk analysis, allowing risk and anomaly detection to be more fine-tuned after the customer is onboarded. Customers who might have otherwise been excluded because they were part of a demographic group considered too risky would stand a chance of a more targeted, and fairer, risk assessment
AI helps customer authentication, onboarding, anomaly detection and reporting
In addition to contributing to financial inclusion, AI, and in particular machine learning, can help in the initial identification and verification of customers. This can be done in the context of remote onboarding and authentication, which will help authenticate users through biometrics, detect fake images and spoofing. Once the customer is onboarded, machine learning can help monitor the business relationship, by conducting behavioral and transactional analysis. Unsupervised machine learning can be used to put customers into cohesive groups based on their transactional behavior which will help set better alert thresholds. It can also be used to improve detection of anomalous behaviour, by identifying outlier patterns in the client network.
Machine learning provides a unique opportunity to incorporate existing knowledge on financial crime typologies on suspicious activity quickly into AML/CFT systems. Through network analysis, AI can also help look at entities’ transactional and social links to other entities with suspicious or confirmed adverse characteristics. AI can help identify and quantify entities’ abnormal behavior with respect to peer groups of similar characteristics, and/or with respect to the entity’s own own historical behavior.
This permits more tailored and efficient transaction monitoring. Supervised machine learning can allow quicker and real time analysis of data according to relevant AML/CFT requirements, and assist in alert scoring, for example by focusing on patterns of activity triggering the need for enhanced due diligence. Natural language processing can help identify and analyze new regulatory requirements as they appear, and integrate those requirements into financial institutions’ AML/CFT compliance systems. Automated data reporting (ADR) can be used to make the underlying granular data held by financial institutions available in bulk to supervisors.
The FATF says AI can contribute to greater auditability, accountability and overall good governance; AI can reduce costs and permit human resources to be devoted to more complex areas of AML/CFT. And of course AI can improve the quality of suspicious activity report submissions.
Obstacles to AI adoption
The FATF acknowledges that there are a number of obstacles to AI adoption. One obstacle is the difficulty to integrate new AI solutions into legacy systems. Another is the inability to combine data from different entities and business units under data protection and bank secrecy laws, which in turn prevents AI solutions from being deployed at scale. A major obstacle cited by financial institutions is the concern that new AI-based solutions would not meet regulatory expectations and that supervisory authorities may lack capacity to evaluate the new solutions’ effectiveness. The lack of explainability of black box models is also a major concern, since it undermines the ability to assess the algorithm’s accuracy in identifying suspicious transactions and other illicit activity. One particular concern related to supervised learning is that the system may learn from poorly labeled data, i.e. historical suspicious transactions reported by the financial institution that may include a high number of false positives or other errors. The absence of a reliable ground truth means that past errors will be trained into the machine learning system.
The FATF also cites the difficulty in defining the acceptable levels of machine error. It has been challenging to develop effectiveness indicators and to determine the acceptable level of effectiveness or residual risks for these new tools.
“Defensive box-ticking”
The FATF emphasizes that financial institutions need to make progress on their risk-based approach. Decision making based on inadequate risk assessments is sometimes inaccurate and irrelevant, according to the FATF. The current approach to risk assessments relies heavily on human input and defensive box ticking approaches to risk, an approach that is both inefficient and burdensome.
The defensive, box-ticking, approach of financial institutions is also attributable to authorities’ response to over-reporting compared to under reporting.
The FATF report highlights examples of successful deployment of AI by financial institutions and supervisory authorities. It also highlights actions taken by regulatory authorities to foster innovation, including the FinCEN’s and U.S. Federal Banking Agencies’ Joint Innovation Statement, which encourages AML pilot programs that are designed to test and validate the effectiveness of responsible innovative approaches.
To learn more: see our editorial in Le Monde, and our article on AI based AML solutions and European fundamental rights, and visit the explainable AI for anti-money laundering (XAI4AML) website.
__________________________________________________
By Winston Maxwell, Astrid Bertrand and Xavier Vamparys, Télécom Paris, Institut Polytechnique de Paris