“Denoising” radar satellite images with artificial intelligence (IP Paris)
07 February 2025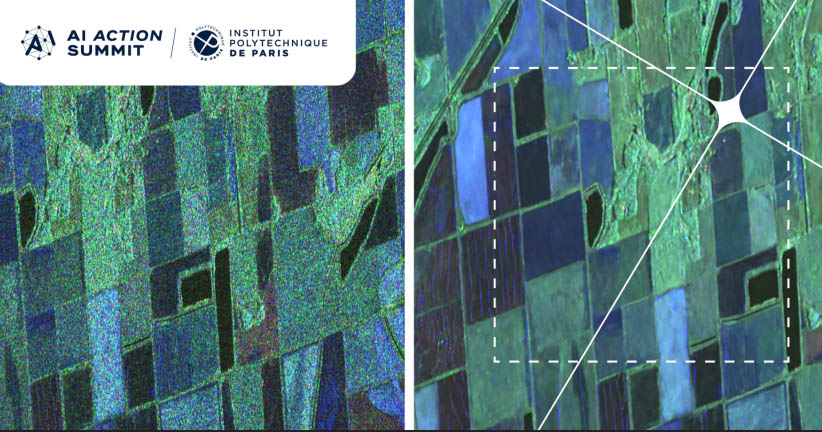
SAR radar image of crop fields, raw (left) and processed by statistical learning (right) © Florence Tupin via IP Paris
“The images obtained have often a lot of fluctuations that result in altered “colours” and a kind of granularity that makes them difficult to interpret,” says Florence Tupin, a professor at the Information Processing and Communications Laboratory (LTCI) at Télécom Paris. The researcher, who specialized in “denoising” these images with the help of artificial intelligence (AI) – more precisely with statistical learning – has developed innovative methods for “drastically improving” them.
In image processing, artificial neural networks – an adaptive AI system that teaches algorithms to process data from a large number of examples – are generally trained to obtain an image without “noise” by showing them the type of image to obtain from the acquired “noisy” image. On the other hand, Florence Tupin and her colleagues’ deep learning approach makes it possible to work without a “ground truth image”. “In short, we provide the network with two noisy versions of the same scene, that is images that present the same information but with different noises, or speckle fluctuations,” says the researcher. “The network will be able to predict the part that is identical to the two images, in other words the scene without noise.”