Scientific report 2013-2018 | 2008-2013 | 2005-2009
The activity of the IMAGES team covers many aspects of the processing, analysis and synthesis of digital images, volumes, and videos. A particularity of the team’s work is controlling the entire image chain, from modeling the acquisition system to specific knowledge of the application domains. Another common point of much of the research carried out by the team is the use of mathematical modeling, with a particular focus on stochastic modeling, optimization and more recently machine learning. Indeed, over the last years, the team’s activity has been marked by a strong shift toward deep learning approaches, with a particular interest in developing methods capable of handling scarce or corrupted data, designing methods for specific imaging modalities, or understanding and structuring generative models. The main application fields in which the team’s activity is focused are: remote sensing imaging, medical imaging, computer graphics, and natural image processing.
Medical imaging
Since 2015, activities in medical imaging have mainly taken a turn toward deep learning (starting with the PhD by H. Bertrand + collaboration with Philips), while maintaining a solid grounding in mathematical models for image analysis and understanding, as well as structural and symbolic approaches. Machine and deep learning methods have been developed for various image analysis and interpretation tasks: reconstruction, registration, segmentation, object recognition, image generation and classification.
One specificity of our work is the development of new methods and deep learning architectures inspired by clinical experts’ reasoning or driven by clinical needs or constraints.
For instance, we introduced topological and geometrical constraints into deep learning models for: 1) vessel segmentation (PhD by A. Virzi, C. Muller and G. La Barbera); 2) increasing the sensitivity of segmentation methods at the extremities of elongated structures, such as the pancreas, which is a notoriously complicated area (CIFRE PhD by R. Vétil); and 3) image registration of images with different topology, such as a healthy image and one with a tumor (PhD by M. Maillard and A. François + Collaboration with St. Anne hospital and MAP5 laboratory).
We also developed new self-supervised, contrastive learning methods to: 1) leverage clinical meta- data, such as age and sex, or known biases (e.g. site-effect, gender) to improve learnt representation (PhD by B. Dufumier, C.A. Barbano, R. Louiset + Collaboration with NeuroSpin, CEA and University of Torino); or 2) take into account multiple, partial and inconsistent expert annotations, which are very common in clinical data-sets (CIFRE PhD of C Ruppli, E. Sarfati). These methods were developed for accurate and unbiased subject-level predictions in different applications (e.g. disease, tumor, lesion).
Generative models were developed to 1) improve transfer learning of segmentation models learnt on (big) adult imaging datasets to (small) pediatric datasets; and to 2) generate missing imaging modalities in multi-modal segmentation (PhD by G. la Barbera). Generative models were also developed for style transfer and neural rendering (PhD by R. Kips + collaboration with L’Oréal).
New representation learning models were also developed to 1) distill the knowledge of a multi- modal segmentation model towards a single-modal one (PhD of M. Maillard) and 2) separate pathological from healthy patterns using contrastive analysis (Post-doc F. Carton and PhD of R. Louiset). Multi-modal segmentation was also addressed for liver and liver tumor detection and segmentation, when images are paired but not registered, with a method to enforce a constraint of similarity of predictions into learning, along with a method to interpret medical image segmentation networks (PhD by V. Couteaux + collaboration with Philips).
Our work on the modeling of spatial relations was pursued, on the one hand by studying to what extent neural networks implicitly use these relations to recognize objects in particular spatial configurations (PhD of M. Riva + Collaboration with St. Paulo University and PSL- Université Paris-Dauphine), and on the other by integrating them into logical reasoning formalism for spatial reasoning. In addition, work on logic was developed in a much more general framework, proposing abstract logic in topos (collaboration with MICS Lab, CentraleSupélec, and CRIL, Université d’Artois). An emblematic application of this work is the segmentation and recognition of fiber vessels (brain white matter, pelvic nerves) from tractograms computed on diffusion MRI images. The original approaches proposed combine geometric modeling of the fibers (efficiently to facilitate their visualization, PhD by C. Mercier + collaboration with LIX), and spatial relationships to other structures, by modeling anatomical knowledge of these fibers. Individual 3D models of patients are thus constructed, integrating organs, pathologies, blood vessels and nerves, for example, and are used to aid surgical planning (PhD thesis by A. Virzi, C. Muller, G. La Barbera + Collaboration with Hospital St. Anne, Necker Hospital and Philips). This work is now entering a valorization process, in order to foster a more extensive dissemination and adoption.
On the reconstruction side, collaborations with the Gordon Center in Boston led to new methods for 1) accelerated dynamic MR imaging using linear and non-linear machine learning-based image reconstruction (PhD of Y. Djebra), and 2) improved brain PET quantification using super-resolution and non-negative matrix factorization (PhD by Y. Chemli). New deep learning methods have also been designed for 3-dimensional reconstruction for breast tomosynthesis, including uncertainty estimation (PhD by A. Quillent, with General Electric). Furthermore, we also launched new collaborations on AI for new segmentation application do- mains, such as histopathology images (PhD by A. Pirovano with KeenEyes, PhD by A. Mammadov with St Joseph hospital, PhD by A. Habis with Institut Pasteur and MSc project by S. Naik with Imperial College London), OCT and spine modeling (PhD by S. Ebrahimi with Arts et Metiers ParisTech). We pursued our activity in biological imaging with compressed-sensing methods applied to fluorescence microscopy denoising (PhD of W. Meiniel with Institut Pasteur) and OCT acquisitions (PhD of W. Meiniel with Columbia University). We continued our collaboration with Columbia University on very large cohorts of lung images to phenotype emphysema using machine learning. Finally, we initiated a number of collaborative projects on novel biological image computing challenges such as neuron tracking on fluorescence imaging of live animals (PhD of R. Reme with Institut Pasteur), or factorial decompositions of uncalibrated bioluminescence images (PhD of E. Dereure with Institut Pasteur). These two recent initiatives led to new collaborations within faculty members of the IMAGES team.
Remote sensing
Activities in remote sensing have been mainly devoted to SAR (Synthetic Aperture Radar) imaging and also from 2021 onwards to hyperspectral imaging. For SAR imaging, three main topics have been investigated: speckle reduction, segmentation and classification, and 3D reconstruction.
Major developments have been proposed for speckle reduction using deep learning frameworks and exploiting the physics of SAR acquisition systems or the multi-temporal potential of satellite sensors (PhD by E. Dalsasso, PhD by I. Meraoumia, ASTRAL project – ANR ASTRID). Supervised and self-supervised methods exploiting temporal diversity or the complex nature of the data have been proposed. The proposed frameworks have been extended to polarimetric data, interferometric data and multi-sensor inputs. The obtained results are state of the art for speckle reduction and have been acknowledged by two awards (best paper of IGARSS 2021 and 2nd best student paper of EUSAR 2022).
Concerning segmentation and classification, the team was involved in the preparation of the SWOT mission (NASA/CNES satellite) as part of the Algorithm Definition Team and also developed classification methods for lakes and rivers based on linear structure detection and Markovian modeling (PhD by N. Gasnier, in collaboration with CNES). Edge detection with a contrario and deep learning methods adapted to SAR statistics has also been proposed (PhD of C. Liu). As for 3D reconstruction, a major effort has been made with regard to SAR tomography as part of the ALYS project and the PhD by C. Rambour for the reconstruction of urban areas using spatial regularization and specific graph-cut based optimization. For forest applications and in preparation for the BIOMASS mission (ESA satellite), new deep learning based reconstruction methods are being developed (ongoing PhD by Z. Bérenger). Both supervised approaches and self-supervised learning through equivariant imaging have been proposed. Multi- view reconstruction based on radiometric information is also currently studied through NERFs approaches.
We also worked on a related topic in a CIFRE PhD with Valeo on deep learning for radar data exploitation of autonomous vehicles (PhD of A. Ouaknine).
Concerning hyperspectral imaging, deep unrolling methods have been explored to propose new interpretable hyperspectral unmixing methods. In this context, auto-supervised training strategies have been considered by simulating the required training sets automatically from the considered data (ongoing PhD by R. Hadjeres).
The topic of multi-temporal analysis of remote sensing images and change detection has been investigated both for optical data (PhD by R. Daudt with the release of datasets for change detection benchmarks and the development of weakly supervised methods and robust to domain shift) and SAR images (PhD of W. Zhao). Work on cloud detection with texture synthesis based on physically constrained generative networks has also been developed. The SAR activities are supported by ANR ASTRID projects (ALYS 2016-2020, ASTRAL 2022- 2026), CNES fundings, Futur and Rupture Fondation Mines-Télécom fundings, AID funding and CSC (Chinese Scientific Council) PhD funding. Collaborations are led with national partners (IETR Université de Rennes, CNAM, MAP5, CESBIO, ONERA) and international academic partners (Tromso University Norway through COSMIC project, Universität der Bundeswehr München through Bay-France project). In addition to publications, the developed methods are accessible as open-source code on the RING (Radar Imaging Group) gitlab https://gitlab.telecom-paris.fr/ring/
Computer graphics
The team has been making notable contributions to 3D and even 4D data manipulations while addressing a wide variety of research and development problems for efficient and effective digital content creation. In rendering, which is a crucial topic in digital image synthesis, a novel material morphing method that preserves details of two material textures has been proposed and a multi-scale rendering technique for dense dynamic stackings such as sand has been developed.
In image synthesis, having the necessary means to model and manipulate 3D geometry is essential. To this end, a high-quality implicit surface reconstruction algorithm, a spectral-preserving mesh simplification, a free-form deformation method using cages, and a parametric shape manipulation method using direct acyclic graph have been proposed.
In creating computer animation, which involves one additional degree of freedom (i.e. time) on top of the spatial dimensions, the team has focused on the physics-based simulation approach. In particular, stable, energy-preserving, efficient simulation methods for soft bodies and fluids have been developed.
A unique specialty of computer graphics is its connection to the arts. The team has demonstrated its expertise in a sketch-driven approach. Instead of relying on 3D representation, artistic manipulation of 2D images and geometries has been developed.
Moreover, thanks to the team’s expertise in both computer graphics and machine learning, the team has been carrying out research into applying machine learning techniques to computer graphics problems. This includes, among others, texture mapping and physics-based simulations, which result in superior methods to the traditional ones.
Shape Modeling
The last orientation of our team’s research activities is focused on natural images and includes works at the interface between image processing, com- puter vision and computational photography. Over the last years, our activity has mainly shifted toward deep learning approaches, with a strong interest in generative models.
A first recurrent area of interest lies in the understanding and structuring of latent spaces of generative models. We have investigated in detail the inner working of simple architectures dedicated to elementary geometric shapes, shedding some light on the way networks handle geometric attributes. In another work, a generic method for structuring the latent space of autoencoders is proposed, taking inspiration from PCA decompositions. Our team also took interest in the structuring of latent spaces for the specific task of face synthesis and editing. Methods have been proposed for face aging, for computing spatially varying editing direction in the latent spaces of StyleGan-like architectures or building intrinsically disentangled latent spaces and for the editing of videos of animated faces.
Another important activity field for our team is texture modeling and synthesis. Here too the main framework is deep generative models. We have significantly improved the state-of-the-art optimization-based approaches to texture synthesis through the careful development of spectral losses and multi-scale schemes. In partnership with Onera, we have developed synthesis methods dedicated to cloud field synthesis and super-resolution, both for visual images and for images of physical properties such as ice or water content. These works build on classical GAN (Generative Adversarial Networks) that are revisited to take into account fractal properties of cloud fields as well as other specific statistical constraints. We have also developed a generic method for the universal synthesis of visual textures, leveraging an autoencoder with self-similar properties and the ability to control long range statistics.
Significant effort has also been devoted to learning strategies for deep architectures. In the field of cultural heritage analysis, we have developed semi-supervised methods for iconographic element detection, adapted to databases that are only lightly annotated. In the same field, we have also investigated transfer learning approaches for painting classification tasks. More recently, we have developed a fully synthetic learning strategy for image restoration tasks, where databases of natural images are entirely replaced by synthetic images, with geometric as well as color priors. To the best of our knowledge, this work is the first to show the feasibility of using synthetic training for real-world restoration tasks.
The team also has a long term expertise in patch-based image and video synthesis/inpainting methods. Recently, this expertise has evolved toward internal learning-based methods, including efficient attention mechanisms inherited from patch-based algorithms and single-image diffusion models for image and video inpainting. To the best of our knowledge, our models are the first diffusion models making it possible to inpaint and synthesize complex videos.
Our work on the automatic assessment of the aesthetic qualities of photographs led us to develop an inventory of the current state of research in this field and to show that, if techniques based on deep networks currently allow a suitable evaluation of objective aesthetic properties, no operational method as yet (based on recommendation methods, social networking or online tests) allows an evaluation of the specific subjective qualities for a given observer.
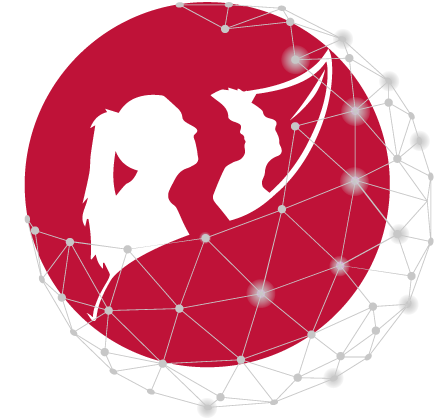